Human + AI. Or how artificial intelligence is being integrated into the lives of creators and beyond
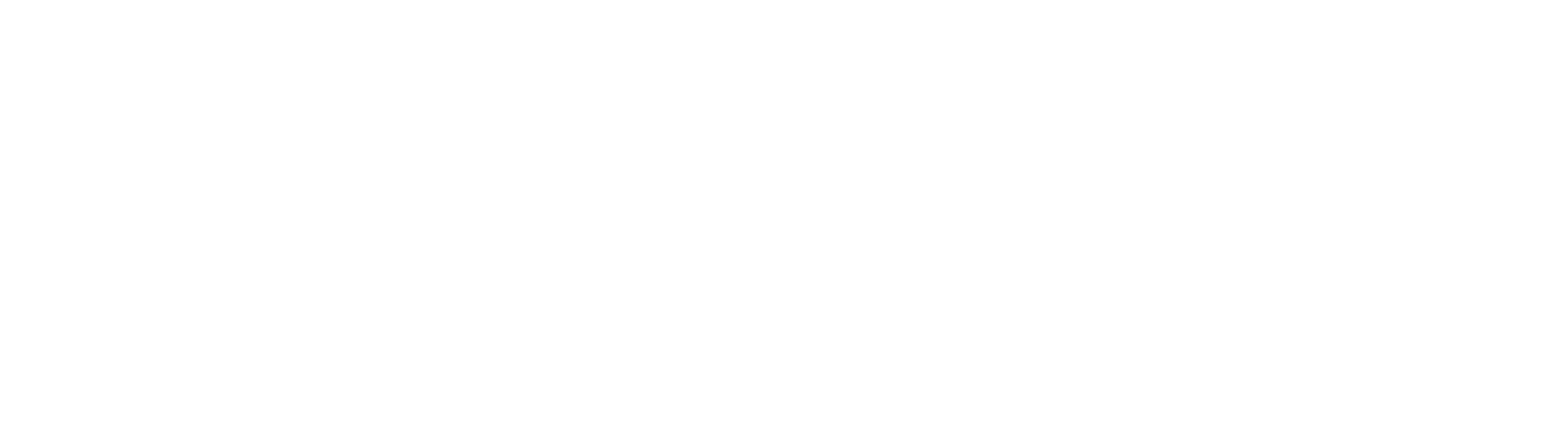
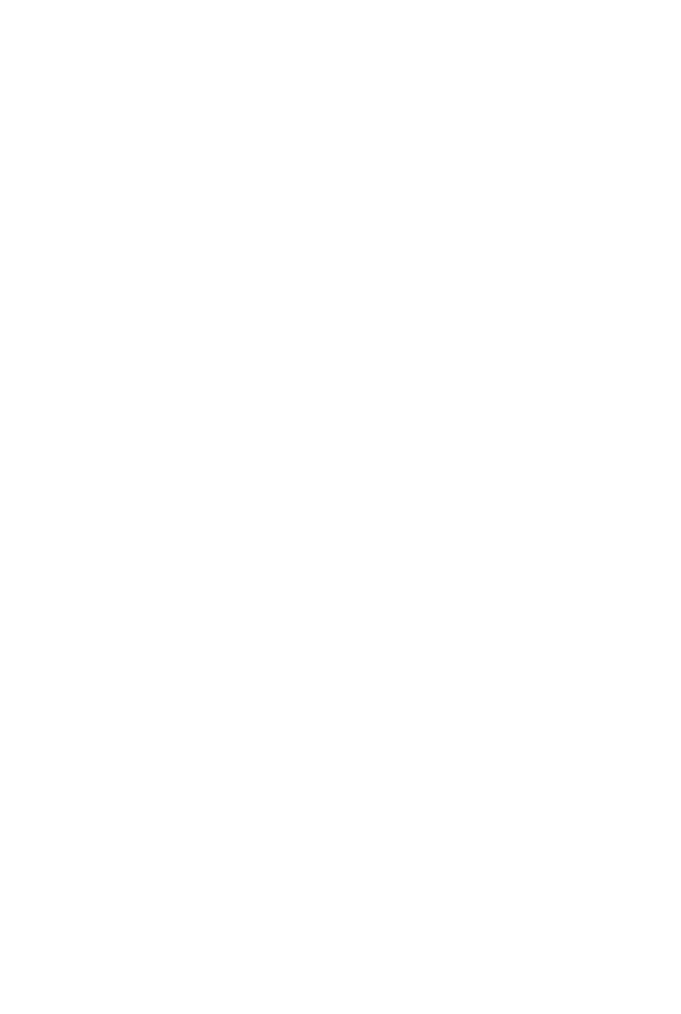
Contents
1. What's going on here?
2. GANs
3. DeepDream
4. CLIP
5. DALL-E
6. Stable Diffusion
7. Midjourney
8. ChatGPT
9. 2023
10. AI makes a fuss?
11. Is there a way out?
12. Summing up
2. GANs
3. DeepDream
4. CLIP
5. DALL-E
6. Stable Diffusion
7. Midjourney
8. ChatGPT
9. 2023
10. AI makes a fuss?
11. Is there a way out?
12. Summing up
What's going on here?
In the next few years, the artificial intelligence market plans to skyrocket. According to UBS Global Wealth Management forecasts, the annual growth rate will be 20%, and the market value will reach $90 billion by 2025. And ChatGPT (a popular AI tool) already hit 100 million monthly active users within two months of launch.
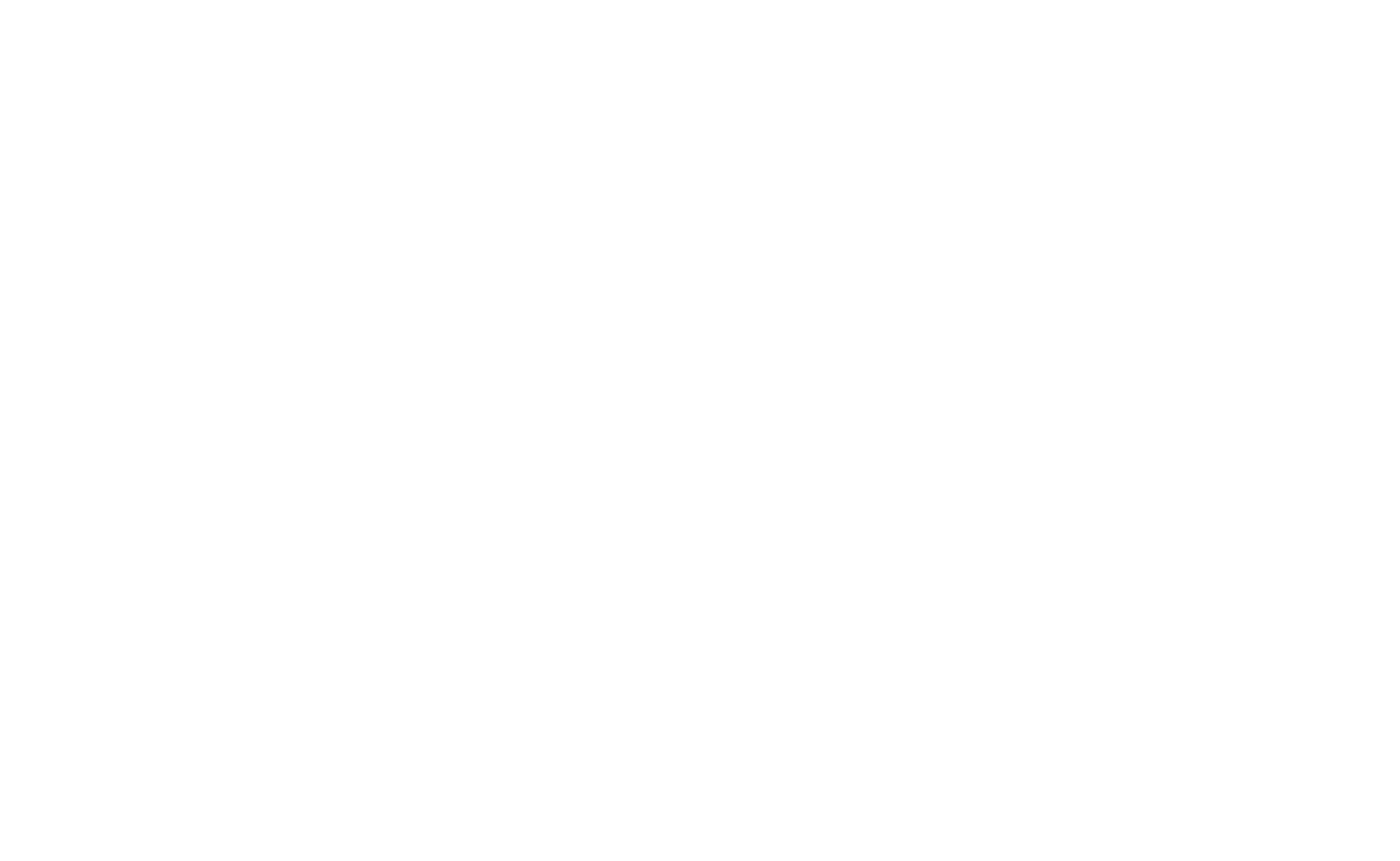
Seeing such prospects, it became interesting for me to explore the possibilities of our interaction with AI. In the article I will tell you about everything that I learned about the emergence of artificial intelligence, and reflect on the validity and groundlessness of disturbing thoughts that we will all be replaced soon.
How was it before 2023, when the AI boom happened? Let's go over the chronology of the development of events in order to know our "enemy" in person and understand how many such "enemies" we currently have in general. There will be no mathematical equations, you can exhale.
GANs
It was 2014. For the first time, they started talking about GAN (Generative Adversarial Network or generative adversarial neural networks). The neural network was introduced by computer scientist researcher Ian Goodfellow and his colleagues. They published a paper describing the concept of GAN and demonstrating its effectiveness in creating realistic images.
Initially, the neural network trained on a large number of different images and remembered distinctive features, such as the faces of people, animals, cars, etc.
The whole essence of the work of GANs lies in its two components: a generator and a discriminator. The generator is trying to create a realistic image, and the discriminator acts as if a critic who is trying to understand: is this a fake or a real image? Due to the presence of a discriminator, the generation of GANs looks very realistic.
The evolution of this neural network is StyleGAN. This is a development from Nvidia, which was released in 2019 and has become widely used to generate content in games and movies. Nvidia, by the way, is still making a good contribution to the development of neural networks for video transformation.
DeepDream
This is a computer vision program from Alexander Mordvintsev from Google. It allows you to create unique and abstract visual images that can be perceived as surreal and fantastic. This technique has aroused great interest and has become popular among artists, designers, as well as computer art enthusiasts.
The idea of DeepDream is to transmit an image through a CNN (Convolutional Neural Network). Then optimize and change the original image to activate or enhance certain patterns and shapes that the model has discovered in the course of its work.
CLIP
With the advent of the CLIP model developed by OpenAi in 2021, we were able to use text queries to control the image generation process.
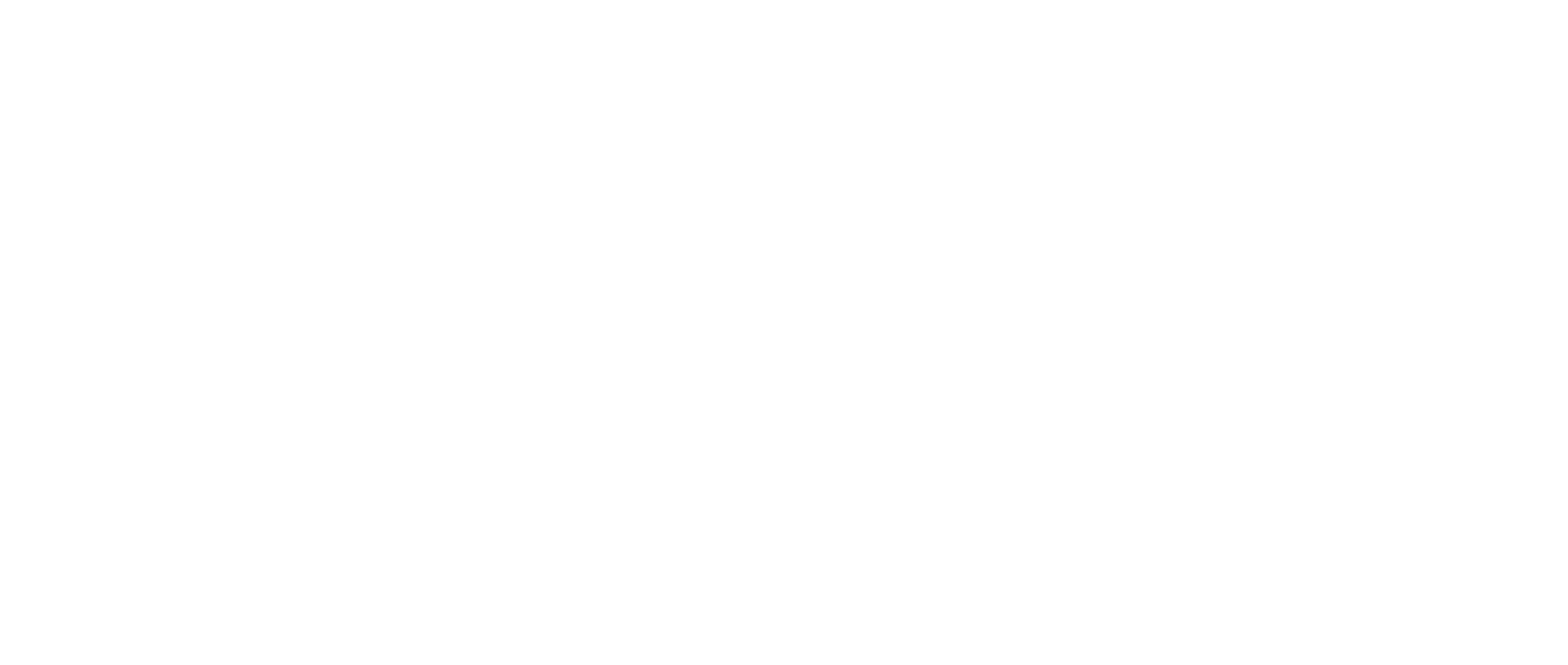
The model parses and associates textual descriptions with images using an approach called "contrast learning". In this way, CLIP has learned to understand the relationship between text and images without the need for a large amount of marked-up data.
DALL-E
The model parses and associates textual descriptions with images using an approach called "contrast learning". In this way, CLIP has learned to understand the relationship between text and images without the need for a large amount of marked-up data. OpenAi made a fuss not only with the release of CLIP, but also with a new tool for generating images - DALL-E. We now have the ability to use text prompts to describe desired features or scenes. For example, you can use the text prompt "pancakes on the table" or "cat in space" and get the corresponding images.
DALL-E is supported by two main components: VQ-GAN (a new development based on the GAN concept) and GPT (Generative Pre-trained Transformer). This is how the whole process of interaction between the two models can be described:
1
GPT takes a text description and converts it into a hidden state vector, a special numeric code that contains information about the text description. That is what the model understands.
2
This vector is then passed to the VQ-GAN image processing model, which decodes it and generates the corresponding image.
In 2022, DALL-E added new features, Outpainting and Inpainting, which immediately distinguished the neuron from its competitors.
What is Outpainting and Inpainting?
Outpainting helps to "finish" the image by expanding its scope. According to OpenAI: "Outpainting takes into account the existing visual elements of an image: including shadows, reflections, and textures to preserve the context of the original image." Inpainting, thus, helps to generate the missing parts, but already inside the image itself. Thus, we can create new compositions by changing objects in the image, or by removing them altogether.
Stable Diffusion
The model became known thanks to the company Stability AI in 2022. It still influences the development of applications related to photo processing, animation, video editing, etc. The word "Diffusion" in the name of the neuron is not in vain, it uses diffusion when generating images.
Briefly, this entire generation process can be described as the development of photographs. In more detail, the generation process consists of the following steps:
1
Selecting an initial image. Could be random noise or any other picture.
2
Diffusion steps. The image goes through several diffusion steps, each of which consists of 2 stages: "blur" and "update".
- The “blur” step results in a blur effect (logically) and reduced image detail.
- "update" step: the model takes the blurry image and generates an updated version that should be sharper and more detailed.
- The “blur” step results in a blur effect (logically) and reduced image detail.
- "update" step: the model takes the blurry image and generates an updated version that should be sharper and more detailed.
3
Lots of steps. The diffusion process is repeated several times (usually hundreds or thousands) to gradually improve the image at each step.
4
Completion of the process. Upon reaching the last diffusion step, we get the final image.
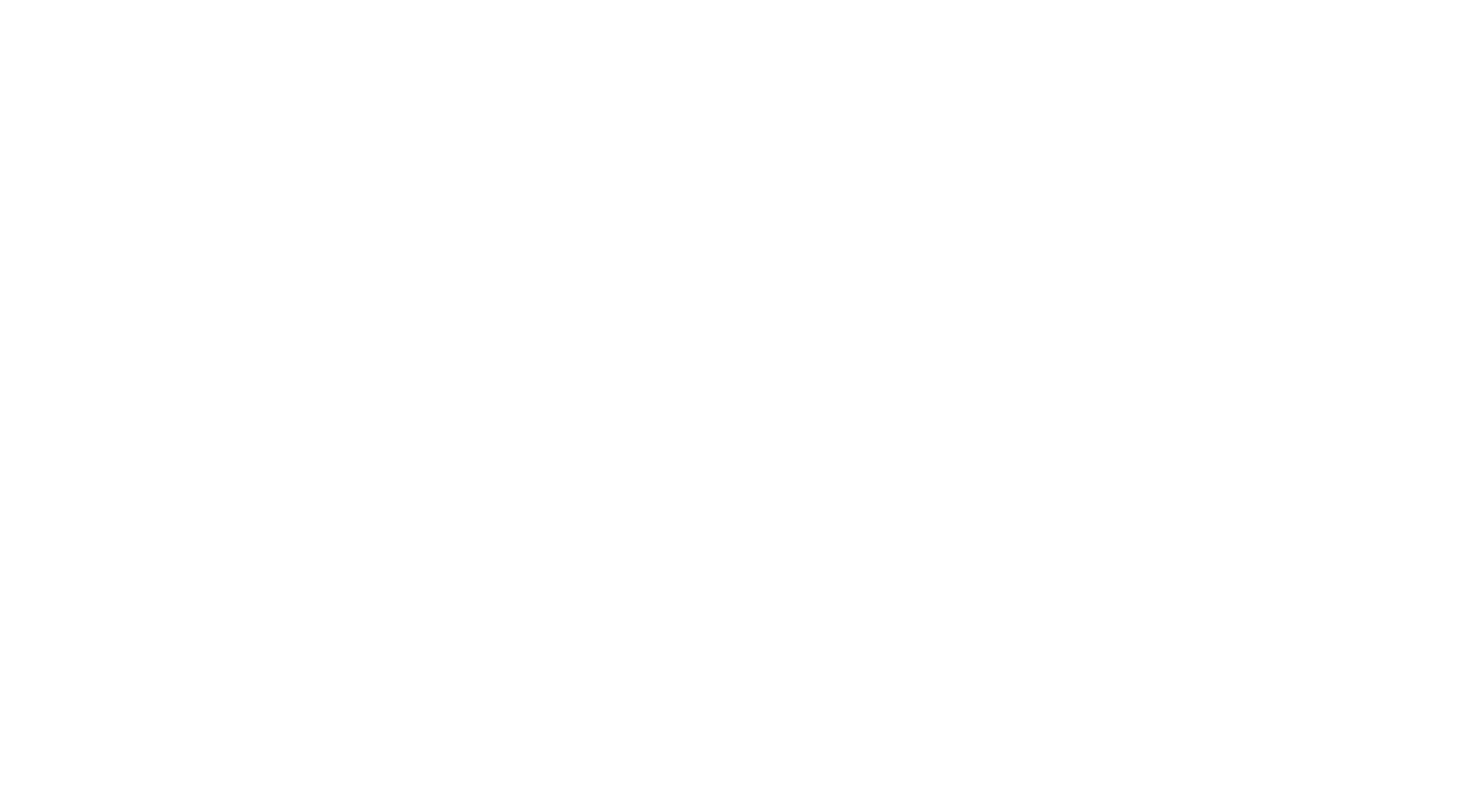
Stable Diffusion is interesting not only because it generates rich, realistic images, but also because it is the only completely open source image generation model, unlike DALL-E and Midjourney (which I will discuss later).
Midjourney
Let's move on to another leader that appeared in 2022, thanks to the independent research laboratory Midjourney, Inc. Let me remind you that, unlike Stable Diffusion, Midjourney is closed source.
The neural network quickly attracted attention, thanks to especially beautiful generations. Their secret is quite simple: the creators of Midjourney opened up access to the results of generations for all users and, thereby, allowed the latter to rate the final images through the Discord interface. Thus, more and more people took part in Midjourney's "training" and this allowed her to level up faster and understand what exactly we like as people.
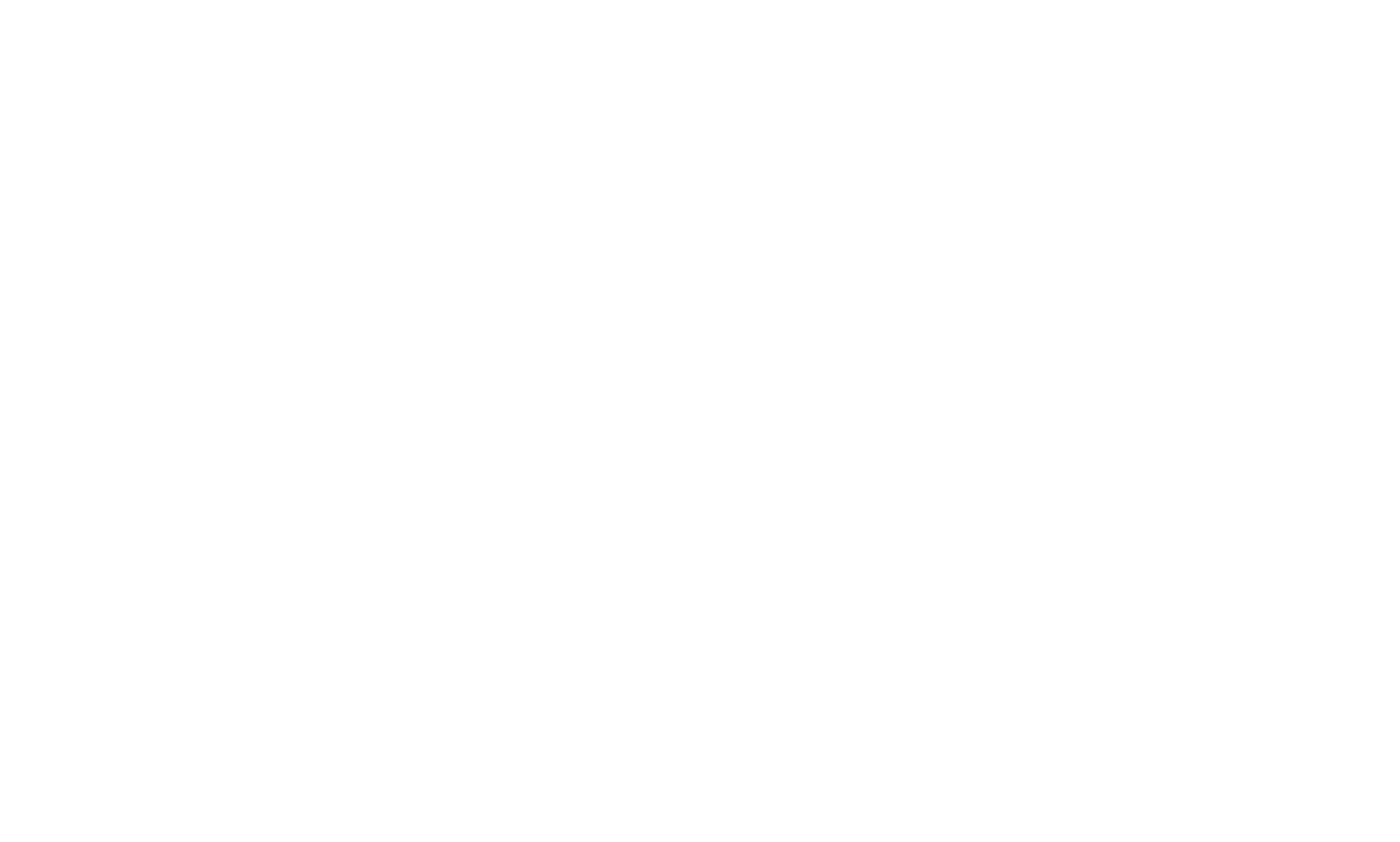
At the moment, 5 versions of Midjourney are already available. But taking into account 5.1 and 5.2, which have their own characteristics, we can say that there are already as many as 7 of them. Unlike DALL-E, we can use previous versions, and unlike Stable Diffusion, these versions really differ in degree of realism from each other.
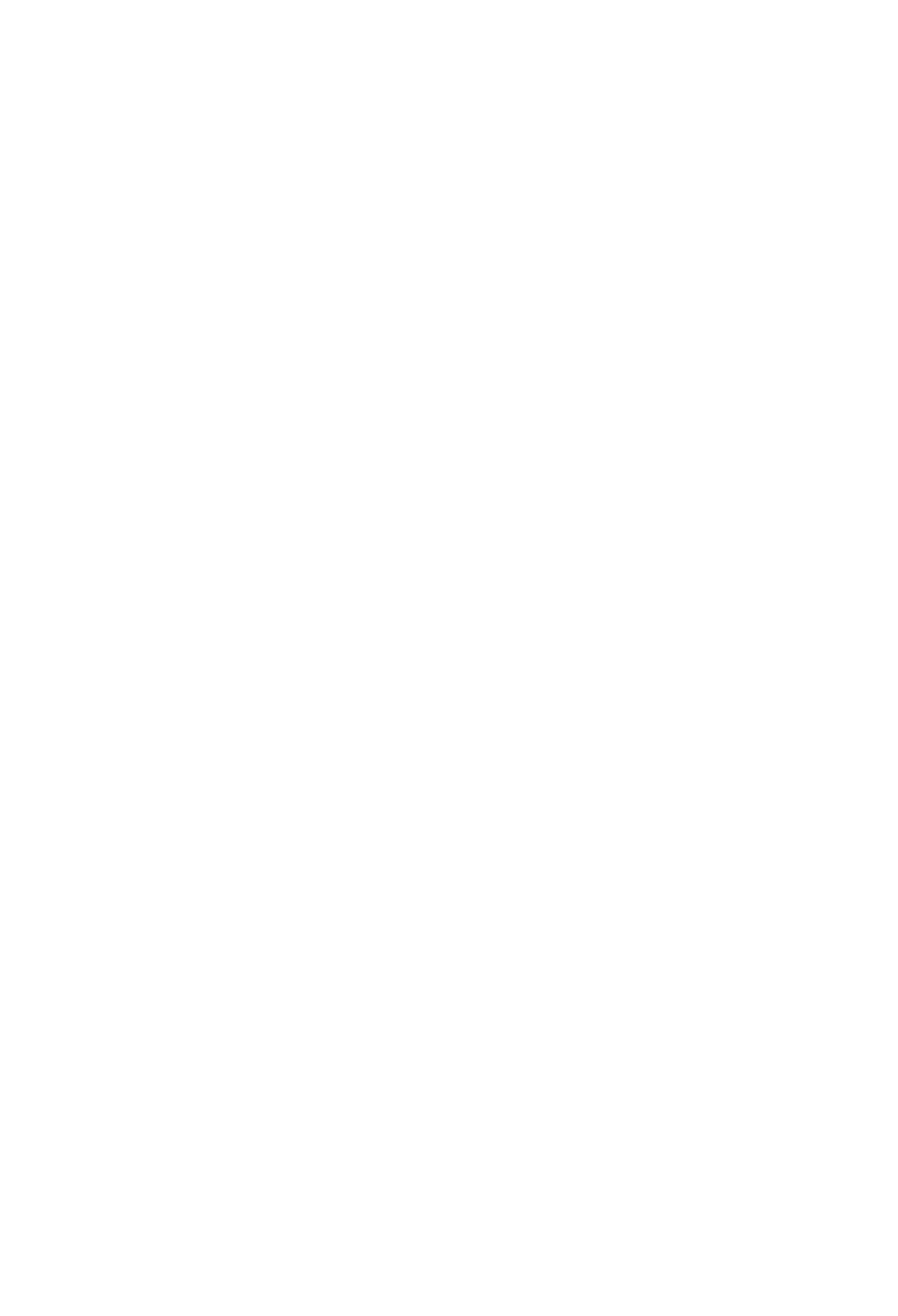
ChatGPT
Although initially I wanted to focus only on neural networks for generating images, I still cannot but mention one universal soldier. What am I talking about? Of course, about ChatGPT. It is part of the GPT (Generative Pre-trained Transformer) family of models that are used to generate text. ChatGPT was introduced as a free neural network chatbot in November 2022 by OpenAI.
ChatGPT already helps with many tasks:
Writing the text, working with its style
Composing slogans
Correction of errors in the code, or their search
Can become a coach for you: help edit your resume, help with learning English
And then as many points as your imagination allows
However, for this you need to be able to communicate with him in the language of prompts. For example, there is already a fairly extensive database of chat prompts that will help you turn him into someone in the right role.
For a long time, the information that the free version of the chat operated on was limited to 2021. You can often encounter misunderstanding if you ask a neuron about the events that happened in 2022.
Here, for example, the chat could not understand what Migjorni is:

However, paying users have gained a significant advantage when using the ChatGPT app for iOS. You can now access information collected by the Microsoft Bing search service.
2023
And again we have come to the starting point - 2023.
Large companies are introducing AI into more and more new services:
Adobe and Firefly's neural network for generating images.
Microsoft and AI graphics tool Designer, as well as Bing and ChatGPT 4 in the search bar.
Google and AI assistant Bard, which they plan to implement, or implement in all the company's services.
Yandex and YandexGPT neurochat, as well as Masterpiece AI image generator.
Opera Software and AI assistant Aria, which was recently added to the updated version of the browser.
Sberbank and neural network for generating Kandinsky images.
And many others - the list continues to grow almost daily.
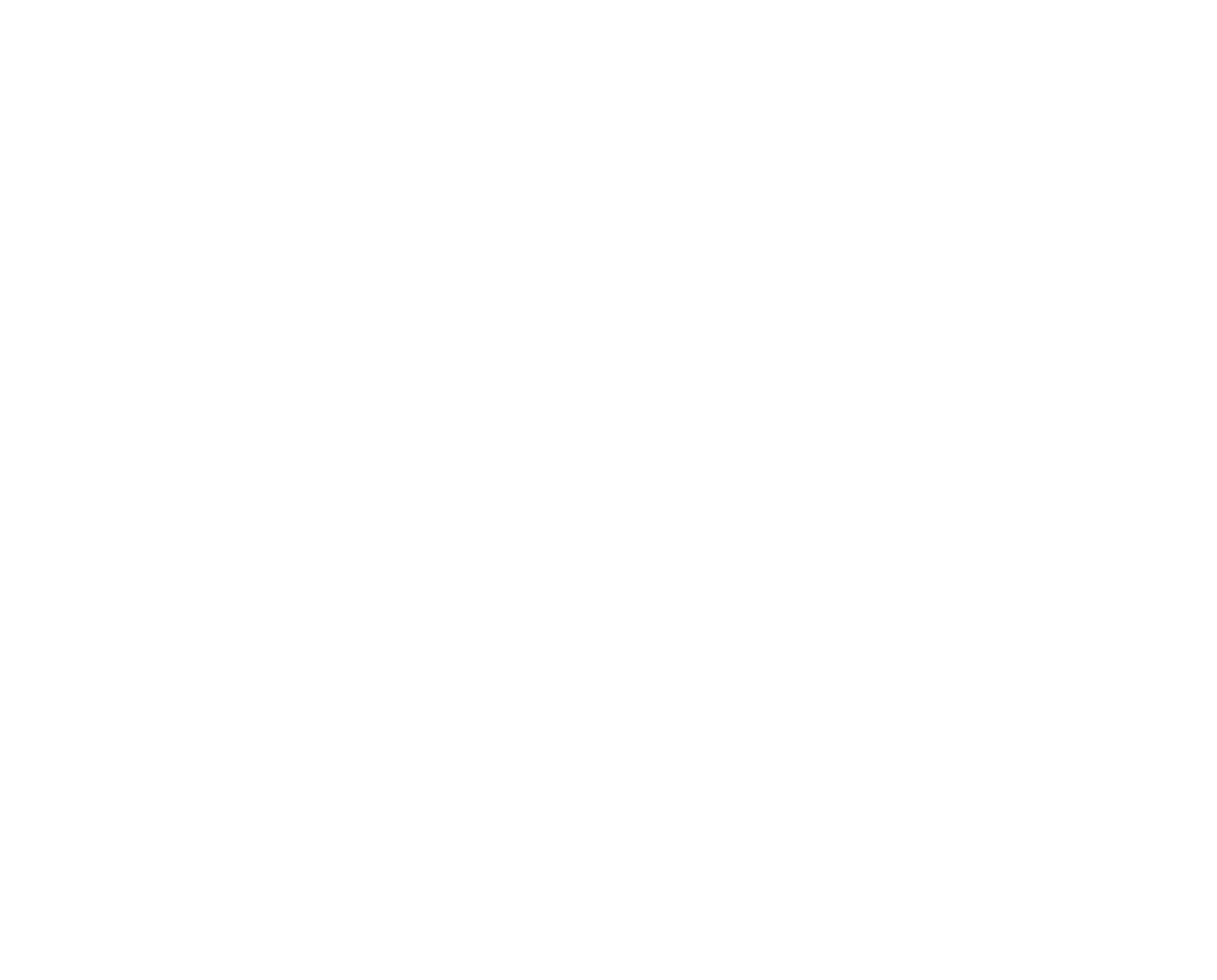
Used in all new areas:
Foodtech
Fintech
Medicine
Industry
Transport
Public administration
And etc
It becomes obvious that artificial intelligence is being introduced into our lives deeper and deeper, making it easier and more convenient in many ways. However, there are some nuances, so I propose to consider the negative consequences of the AI boom that we can observe at the moment.
AI makes a fuss?
Everything would be fine, but you can’t do without problems. At the moment, there are three main problems associated with the development of artificial intelligence.
The problem of deepfakes
With the rapid development of neural networks, it is becoming increasingly difficult to distinguish fiction from reality. For example, consider the case when a photo of the Pope in a down jacket made a splash because many took it at face value.
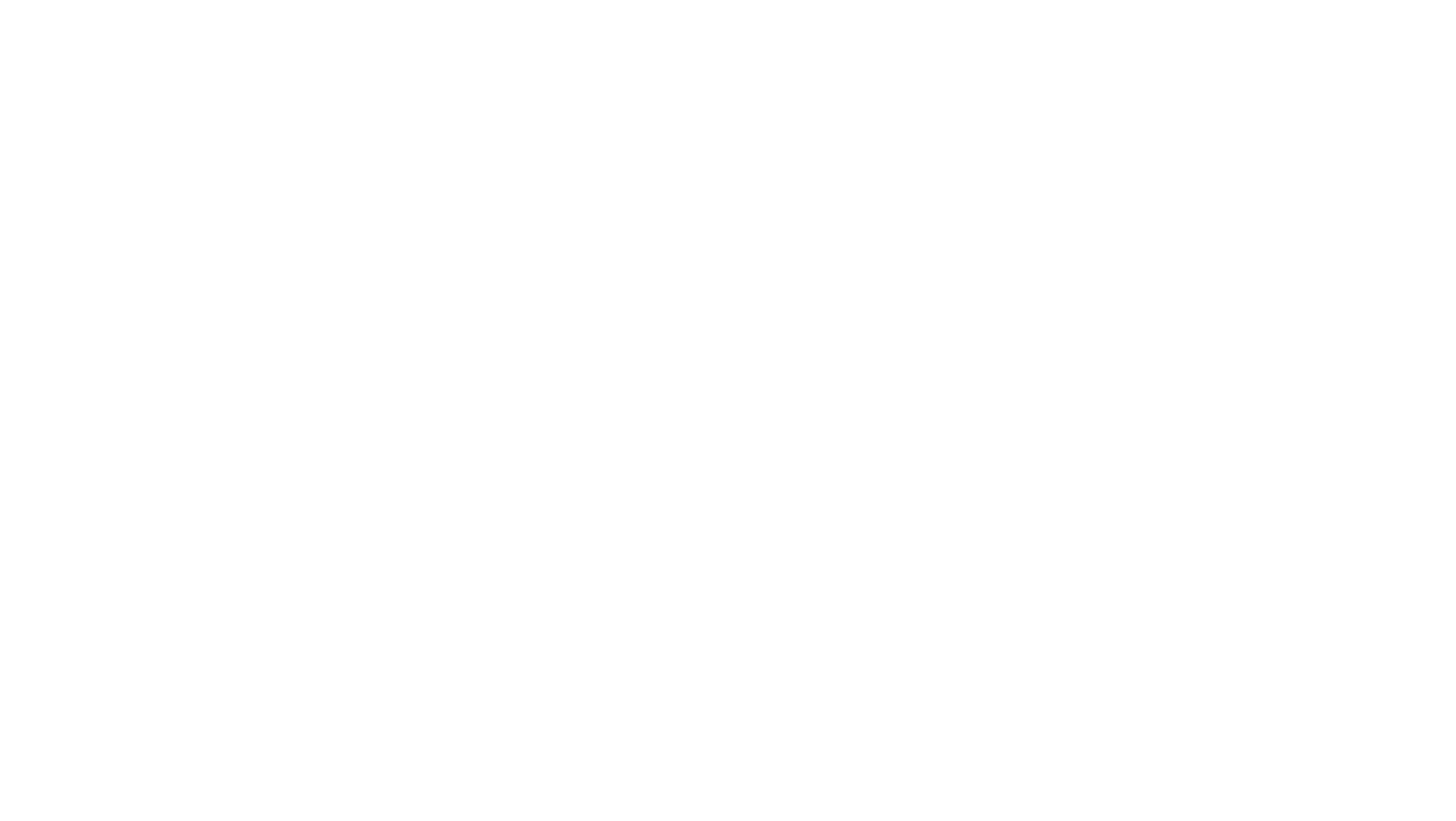
An even bigger scandal erupted over a photo that won a prestigious competition from Sony, but in fact it turned out that it was generated by a neural network.
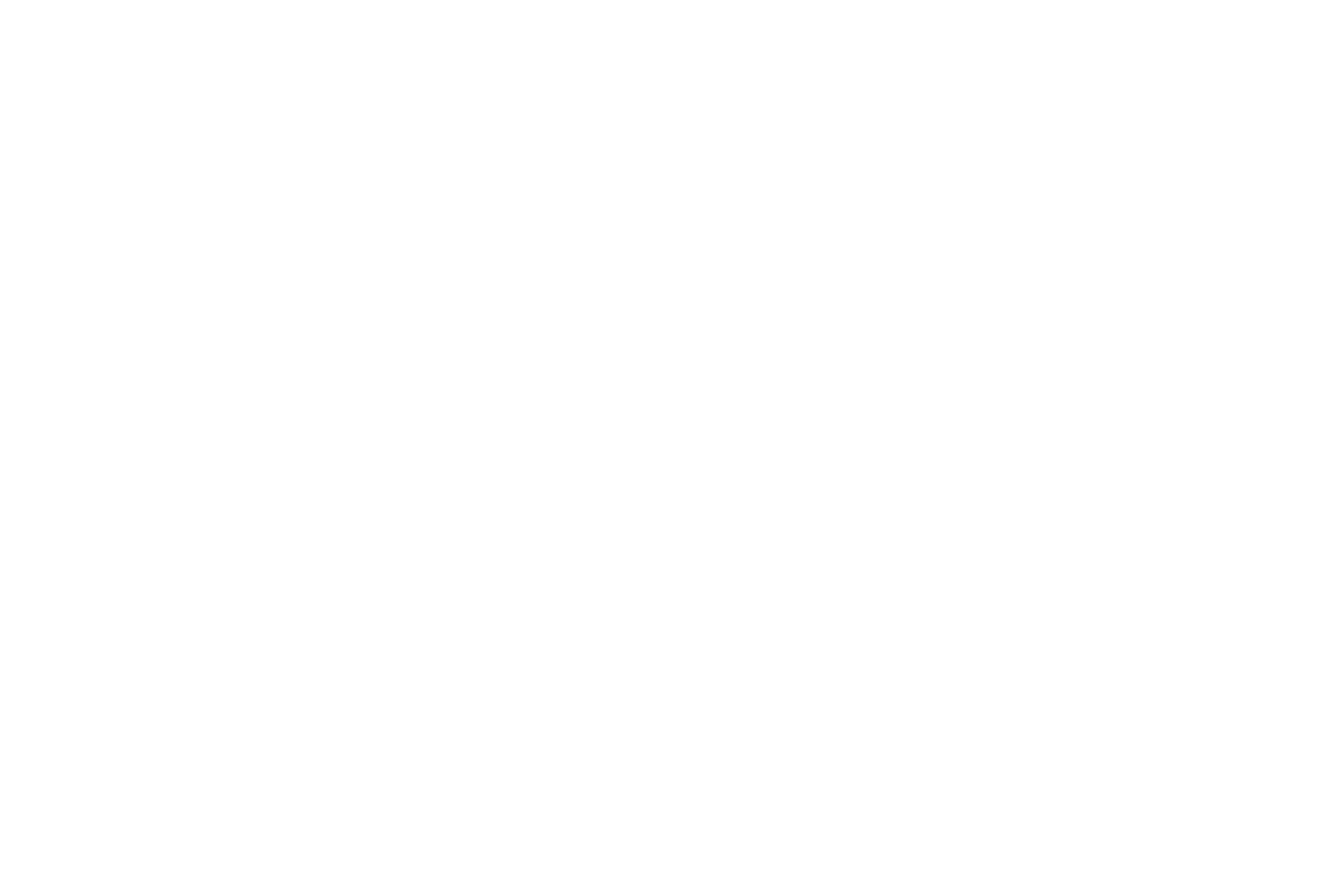
Copyright issue
In its generations, neuron networks often use photographs or illustrations that have already been previously drawn by someone. Thus, watermarks, or copied parts of the originals, sometimes slip through the generations.
Getty, an image licensing service, has filed a lawsuit against the developers of Stable Diffusion, accusing them of illegally using their photos. As a result, 12 million copyrighted images, as well as their descriptions and metadata, were used to train Stable Diffusion. For each image, compensation of $150,000 was demanded, the total amount was 1.8 trillion dollars.
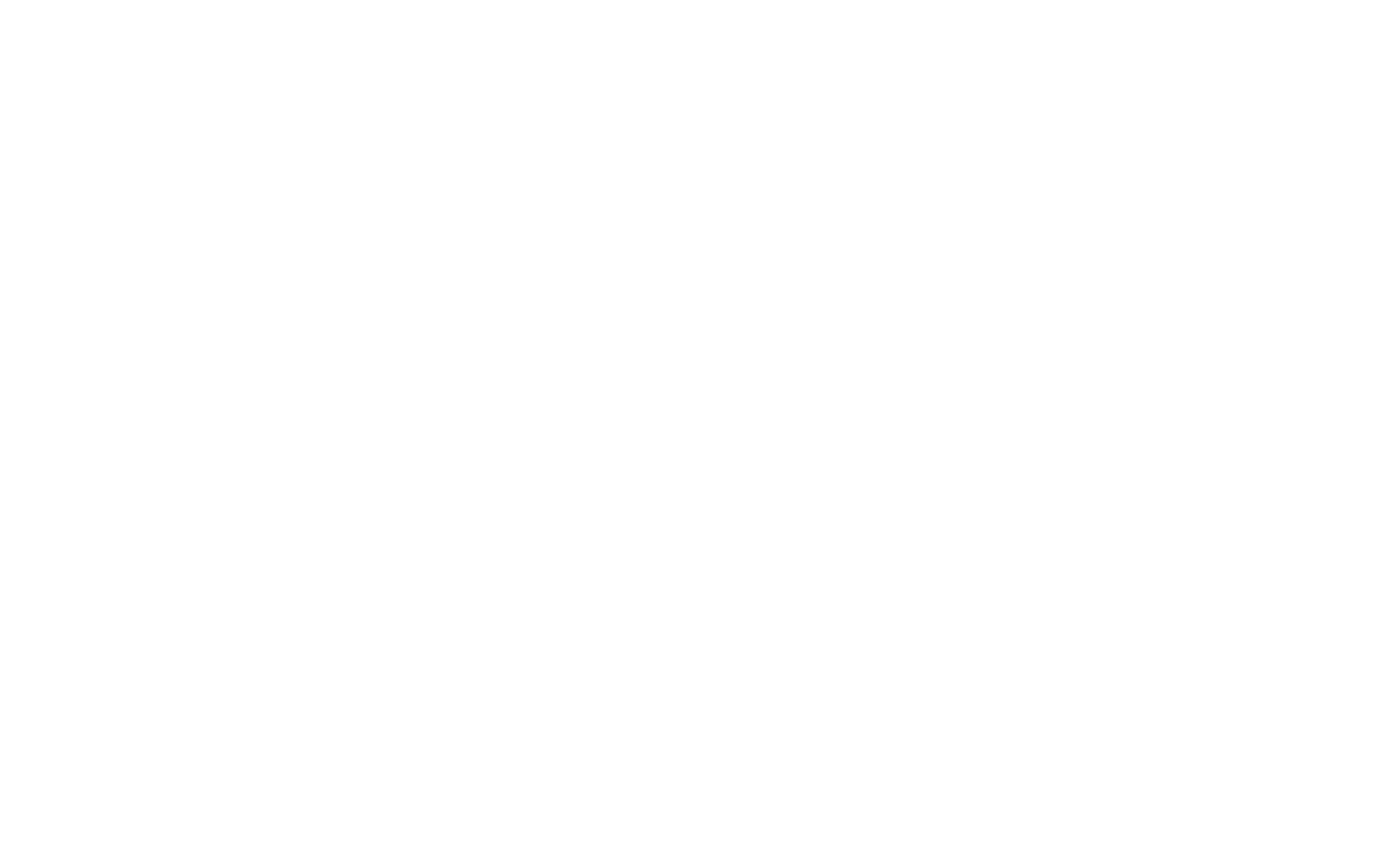
Fake news problem
In addition to celebrity faces, fake news is also in high demand. One explosion near the Pentagon was worth something.
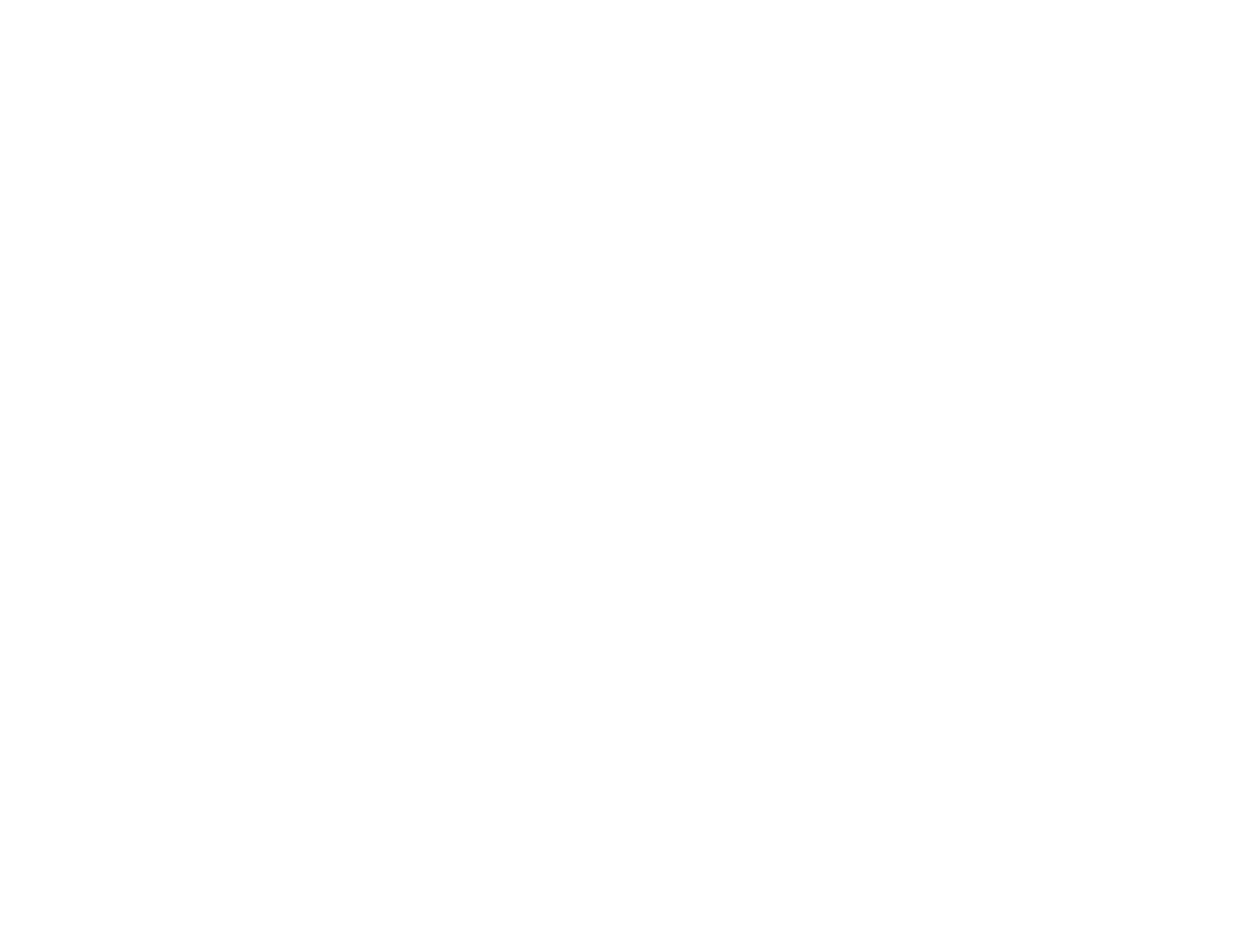
Is there a way out?
We talked a little about the problems, but what about them, and how are we planning to solve them? At the moment, there is no unified system of control over what belongs to whom in the generation of neurons. Just a list of general requirements to:
The developers took measures to ensure the transparency of training models, provided information on what exactly they are trained on.
Creators, in turn, were more actively monitoring the copying of their work.
Companies have taken a closer look at the use of illegal AI creations for their promos.
And one more addition is to think critically and not immediately trust what you see. So we can avoid the spread of the next fake news and images in time. At a minimum, you can follow the following rules for self-detection of fakes:
1
Artifacts. Pay attention to the presence of unnatural textures, repeating patterns, or anomalous details in the image.
2
Comparison. Remember how a particular object usually looks in reality.
3
Context. Study the context in which the picture was found. For example, if it was found on a specialized site for generating images, then you are more likely to have a fake.
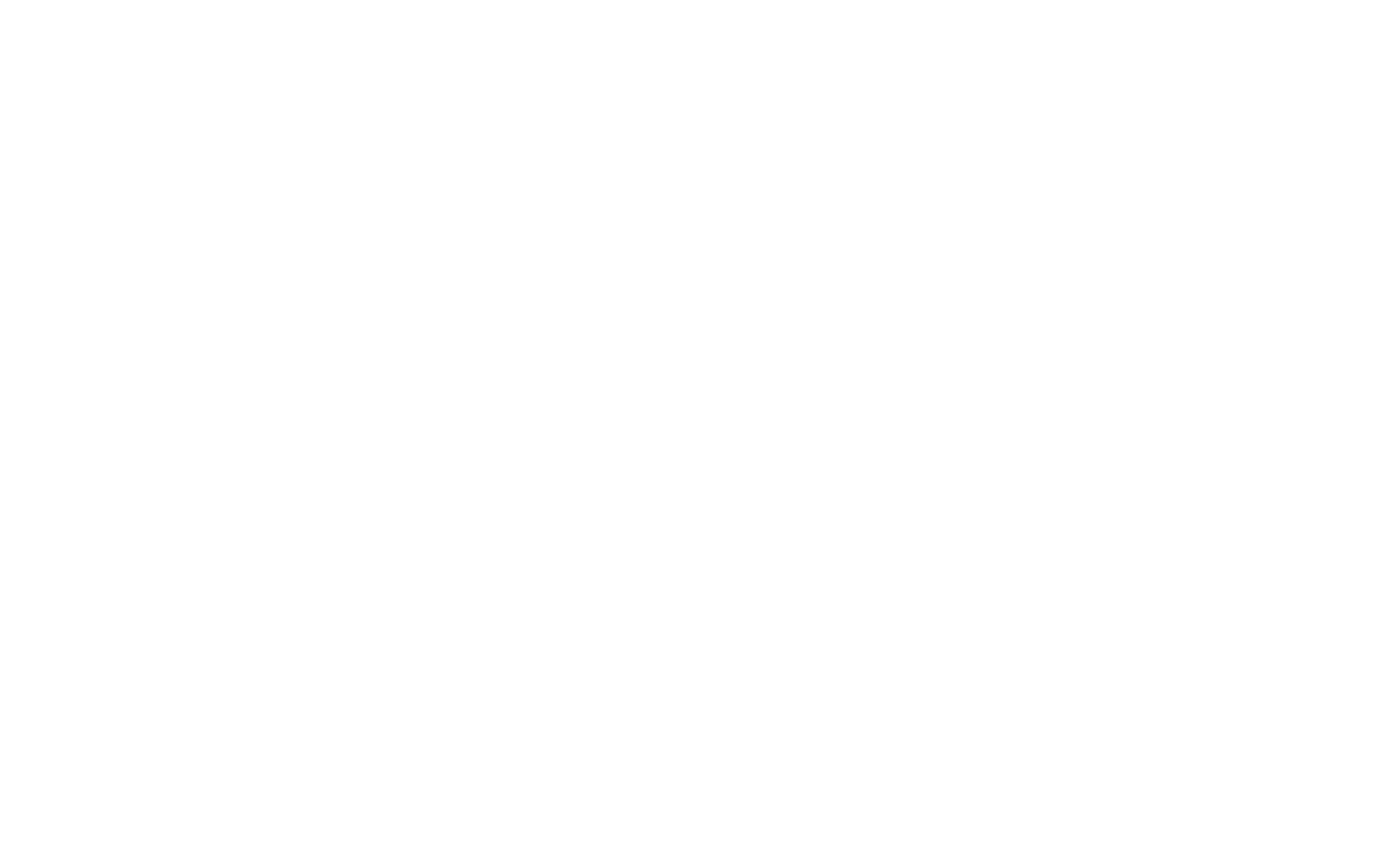
Most likely, in the future everything will come to a clearer regulation of the work of AI. As an option - to the development of a large number of services for searching for borrowed images, recognizing deepfakes, etc. For example, Google is already planning to tag all artificially generated images in search.
Summing up
As you can see, in our 'brave new world,' there is both good and bad. Embracing the development of artificial intelligence can significantly reduce the time spent on various tasks, fostering creativity even when things go awry on some days. As we navigate this dynamic landscape, let us not forget to stay attuned to the release of new, innovative tools.
If that ChatGPT supports my words and has already issued such an example solution from the title of the article:
